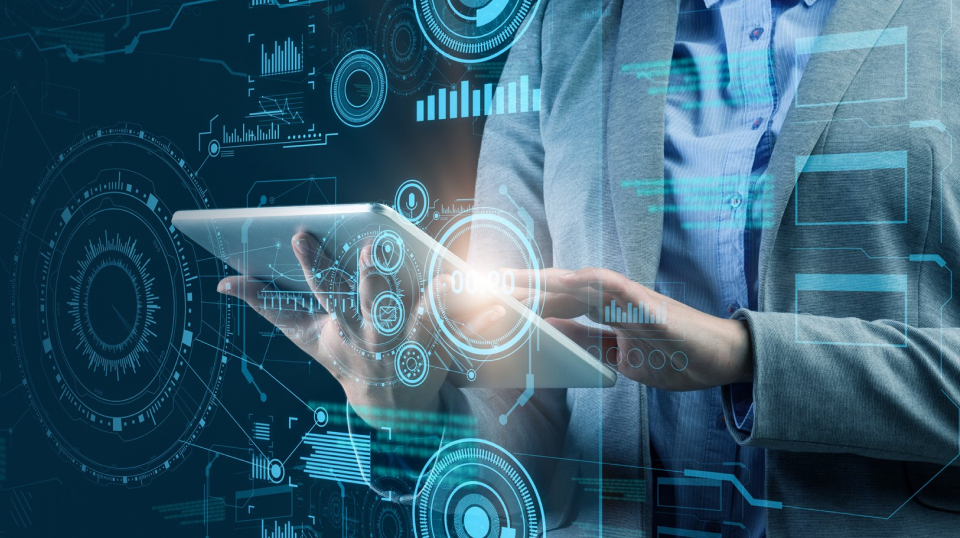
In the digital era, data is the foundation of every strategic decision. It drives decision-making, enhances customer experience, and boosts operational efficiencies. To harness the full potential of data, organizations are investing heavily in data engineering and data management. These two disciplines are crucial for building a strong data foundation, turning raw data into actionable insights that fuel innovation and strategic initiatives.
Data engineering and data management represent the backbone of any modern data-driven strategy. Data engineering centers on designing, building, and maintaining the systems and architectures that facilitate data flow within an organization. Data management, on the other hand, encompasses the policies, processes, and tools necessary to ensure data quality, governance, accessibility, and security.
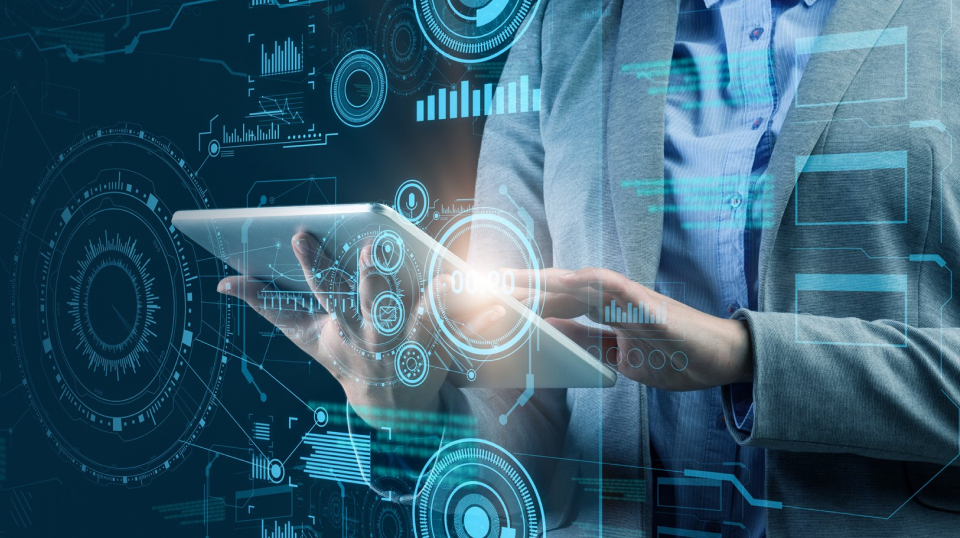
Together, these functions support the overarching goal of digital transformation: leveraging data to make informed, agile, and customer-centric decisions. This blog explores data engineering and data management from the perspective of digital transformation, detailing their roles, key components, challenges, tools, and best practices for organizations aiming to become fully data-driven.
The Role of Data Engineering in Digital Transformation
Data engineering plays a foundational role in digital transformation by constructing the data pipelines and architectures that move data seamlessly across an organization. In a transformation-oriented setting, data engineering enables the integration of disparate data sources, establishes data consistency, and ensures data accessibility, making it easier for organizations to harness insights that drive meaningful business outcomes.
Key Functions of Data Engineering
- Data Pipeline Development : Data engineering entails the design and deployment of data pipelines—workflows that automate the movement of data from one system to another. These pipelines are essential for extracting data from various sources, transforming it into usable formats, and loading it into storage locations like data lakes or data warehouses. Effective data pipeline development underpins all data analytics and machine learning efforts.
- Data Architecture and Infrastructure : As organizations shift towards digital transformation, they need a data architecture capable of managing diverse data sources and formats at scale. Data engineers create the framework within which data flows—typically involving data warehouses, data lakes, or hybrid lakehouses—to store, manage, and analyze vast amounts of structured and unstructured data.
- Data Integration and Orchestration : Data integration is essential for digital transformation since it enables data from various systems to be harmonized. This process often involves integrating data from legacy databases, third-party applications, IoT devices, and cloud platforms. Data orchestration tools automate the scheduling and monitoring of these data workflows, ensuring that data is reliably processed and readily available.
- Real-time Data Processing : Real-time data processing is increasingly critical in digital transformation contexts, where organizations require up-to-the-minute information to drive decisions. Data engineering supports real-time processing by building systems that can handle continuous data streams, allowing for timely insights in areas such as customer experience, operational efficiency, and fraud detection.
- Data Transformation and Enrichment : Data often requires cleansing and standardization before analysis. Data engineering involves transforming raw data into formats that are consistent and usable, eliminating errors, handling missing values, and enriching data with additional contextual information. This step ensures that data delivered to end-users and applications is high quality and ready for meaningful analysis.
Data Engineering Tools
Data engineering tools streamline data collection, ETL (Extract, Transform, Load) processes, integration, and real-time streaming. They support scalable storage solutions and data transformation, creating efficient data pipelines that power analytics and data-driven strategies.
Additionally, these tools facilitate real-time data streaming, allowing data to be processed immediately as it’s generated. Scalable storage options ensure that vast amounts of data are securely stored, while data transformation and preparation tools make it ready for insights and decision-making. Together, these tools create a seamless data pipeline that fuels an organization’s analytics and data-driven strategies.
Understanding Data Management: The Backbone of Digital Transformation
As organizations strive to harness their data effectively, they must address key aspects of data management. These core components form a strong framework for maximizing the value of data assets, ensuring they are well-governed, secure, and ready for analysis. By managing these elements effectively, businesses can ensure that their data is accurate, accessible, and actionable, enabling informed decision-making and driving innovation across various domains.
According to a report by IBM, data management practices are evolving to focus more on compliance and data quality (Belcic & Stryker, 2024).
Core Components of Data Management
- Data Governance
Data governance establishes policies, procedures, and responsibilities for managing data throughout its lifecycle. This process includes defining roles and permissions, setting data standards, and ensuring compliance with regulatory requirements. Effective governance aligns data management practices with organizational goals, ensuring data integrity and accountability.
- Data Quality Management
High-quality data is essential for reliable analytics and decision-making. Data quality management includes profiling, cleansing, and validating data to maintain its accuracy, completeness, and consistency. Poor data quality can erode the trust of stakeholders and lead to faulty decisions, so organizations must prioritize data quality as part of their transformation strategy.
- Metadata Management
Metadata provides context about data, such as origin, structure, and relationships, enhancing its discoverability and usability. Metadata management involves cataloging and organizing metadata, which enables data users to search for and understand data across the organization. Metadata management supports transparency and trust by providing lineage and provenance details about data.
- Master Data Management (MDM)
MDM involves creating a unified and authoritative source of truth for key data entities, such as customers, products, and suppliers. This practice eliminates data redundancies, aligns departments around consistent data, and facilitates smoother business processes. MDM is especially crucial in digital transformation as it ensures that critical business data is standardized and reliable.
- Data Security and Compliance
In a digital-first environment, protecting data from unauthorized access and ensuring regulatory compliance are critical. Data management frameworks incorporate security measures such as encryption, role-based access control, and audit logging to safeguard sensitive data and uphold privacy regulations like GDPR and CCPA. Secure data management practices foster trust among customers and partners while minimizing the risk of data breaches.
Data Management Tools
Data management tools are essential for efficiently storing, organizing, securing, and analyzing data, allowing organizations to unlock its full potential. These tools cover a range of functions, including data integration, quality, governance, and visualization. They support real-time data streaming, ETL (Extract, Transform, Load) processes, and data preparation and blending.
Data governance tools help maintain compliance and data integrity, while scalable data warehousing solutions handle vast data volumes for analysis. Visualization tools further empower organizations to interpret data effectively, enabling impactful analysis and insights. By leveraging these solutions, organizations can ensure their data is accessible, accurate, and actionable.
Overcoming Challenges in Data Engineering and Management
Data engineering and management face a range of complex challenges that organizations must navigate to derive meaningful insights.
- Data Integration and Interoperability: Combining data from diverse systems is complex due to differing formats and protocols. Achieving a seamless flow across systems, especially with older technology, requires strategic integration to support unified data insights.
- Breaking Down Data Silos: Data often exists in isolated departmental silos, limiting a full organizational view. Consolidating this data is essential for cross-functional insights but requires coordination and investment in unified solutions.
- Scalability and Cost Control: As data grows, maintaining fast, efficient storage and processing is challenging. Organizations must ensure their infrastructure scales to handle large data volumes while controlling costs, especially in cloud environments.
- Privacy, Compliance, and Security: With stringent regulations like GDPR, protecting data privacy and ensuring compliance is paramount. Strong data protection measures and policies are necessary to safeguard sensitive data and maintain legal compliance.
- Real-Time Processing: Immediate data insights are crucial for industries that require real-time decision-making. However, achieving low-latency data streaming demands advanced architecture and significant resources.
- Data Lifecycle Management: Efficient data lifecycle management—from creation to archival—helps keep data relevant and minimizes storage costs, requiring effective policies for retention, archival, and purging.
- Ensuring Data Quality: Consistent and reliable data is vital for accurate analytics. This requires robust validation, deduplication, and quality control across data sources.
- Data Governance: Implementing strong governance practices is critical for aligning data usage with organizational goals and compliance requirements. This can be complex, especially with growing data and evolving regulatory standards.
- Fault Tolerance and Resilience: Ensuring systems are resilient to errors and capable of recovery is crucial, especially for critical applications that require high availability.
- Data Discoverability: As data grows, it’s essential to organize it for easy discovery and use. Effective metadata management is necessary to make data searchable and accessible.
- Addressing Skill Gaps: Data engineering requires specialized expertise in architecture, governance, and analytics. Many organizations face shortages in these areas, making ongoing training and hiring essential for strong data practices.
These challenges can be effectively addressed by implementing scalable, customizable solutions paired with expert guidance in data governance and security. With a balanced approach that combines advanced technology, robust governance practices, and skilled personnel, organizations can transform their data into a strategic asset. This comprehensive framework ensures that data management is optimized for resilience, compliance, and adaptability, empowering businesses to drive innovation and achieve sustained growth in a rapidly evolving digital landscape.
Best Practices for Data Engineering and Data Management
To maximize the effectiveness of data engineering and management efforts, at Aventior we adopt best practices that ensure data quality, scalability, and security.
- Build a Scalable Data Architecture
A scalable architecture allows organizations to respond to growing data volumes and changing data needs. Cloud-based platforms, such as AWS, Azure, and Google Cloud, offer flexibility and on-demand scalability, allowing organizations to manage resources efficiently and avoid infrastructure bottlenecks.
- Establish Strong Data Governance
Clear governance policies provide a framework for managing data effectively. Organizations should define roles, responsibilities, and data access permissions while setting standards for data quality, consistency, and compliance. This foundation ensures data integrity and regulatory adherence, supporting trustworthy analytics and insights.
- Prioritize Data Quality from the Outset
Quality data underpins effective decision-making, so organizations should implement data profiling, validation, and cleansing processes at the earliest stages. Automated data quality checks ensure that data remains accurate, complete, and consistent, preventing errors from cascading through systems.
- Use DataOps to Enhance Agility
Adopting DataOps principles—borrowing from DevOps—enables organizations to accelerate the development, testing, and deployment of data pipelines. DataOps encourages collaboration between data engineers, analysts, and business users, resulting in faster insights and more responsive decision-making.
- Embed Security and Privacy by Design
Incorporating security and privacy measures at every stage of the data lifecycle protects sensitive information and helps meet regulatory requirements. Encryption, role-based access, and anonymization techniques provide safeguards without compromising accessibility or usability.
- Foster a Data-driven Culture
Finally, achieving digital transformation requires fostering a data-driven culture across the organization. This involves promoting data literacy, encouraging data-based decision-making, and ensuring that data is accessible to all relevant stakeholders. When data becomes a shared asset, the entire organization is empowered to contribute to transformation goals.
Streamlining Data Management: Aventior’s Smart Solutions for Real-Time Processing and Pharma Efficiency
Aventior’s data engineering services focus on designing and deploying robust data pipelines that automate the movement of data from source systems to storage locations such as data lakes or warehouses. These pipelines extract, transform, and load data, enabling seamless analytics and machine learning workflows. Our solutions include real-time data processing, scalable architecture, and effective integration across diverse data types. Aventior’s visualization expertise further ensures that actionable insights are easily accessible via platforms like Tableau and Power BI.
Aventior’s proprietary platforms, CPV-Auto™ and DRIP, represent innovative solutions aimed at streamlining data management and driving efficiency in the life sciences and pharmaceutical sectors.
CPV-Auto™ automates the digitization and analysis of batch records in pharmaceutical manufacturing, helping organizations adhere to regulatory standards like FDA compliance. By transforming unstructured data into structured formats, CPV-Auto™ enables faster decision-making and operational efficiencies. This platform facilitates improved batch release times, accurate data storage, and real-time access to Critical Production Parameters (CPP) for enhanced process conformance and audit readiness.
On the other hand, DRIP (Data Restructuring and Informatics Platform) addresses the challenge of managing vast and varied datasets generated during drug research and testing. By automating data integration and structuring, DRIP helps pharmaceutical companies consolidate disparate data sources, making it easier to search, analyze, and extract actionable insights. This platform supports the consolidation of extensive pharma, biotech, and life sciences data, enhancing the ability to derive meaningful insights from large datasets. Both platforms illustrate Aventior’s commitment to utilizing cutting-edge technology to simplify complex data processes, ensuring compliance, improving productivity, and accelerating time to market for life sciences companies.
.Both platforms illustrate Aventior’s commitment to utilizing cutting-edge technology to simplify complex data processes, ensuring compliance, improving productivity, and accelerating time to market for life sciences companies.
Conclusion
Data engineering and data management are crucial pillars of any digital transformation strategy. By building robust data pipelines and architectures, Aventior ensures that data is always accessible and ready for analysis. Effective data management practices complement this by safeguarding data quality, enforcing governance, and providing strong security measures.
As organizations invest more heavily in digital transformation, the synergy between data engineering and data management will be key to unlocking sustained, data-driven innovation. Businesses that fully harness the potential of their data are better positioned to adapt quickly, outperform competitors, and seize new opportunities in an increasingly digital world.
At Aventior, we leverage our expertise in data engineering and data management to transform raw data into a valuable strategic asset. Our solutions, powered by platforms like CPV-Auto™ and DRIP, address complex industry challenges, enhance compliance, and deliver actionable insights. By focusing on scalability, seamless integration, and unwavering data quality, we help organizations maximize the value of their data and gain a competitive edge.
Contact Us today to learn how our tailored solutions can empower your business. Our team of experts is here to understand your unique needs and provide customized strategies that drive efficient, impactful results.
To know further details about our solution, do email us at info@aventior.com.